:::-People
Research Fellow (Prof.) Chun-Shien Lu, Academia Sinica
Director/University/Institute
Research Fellow (Prof.) Chun-Shien Lu, Academia Sinica
Research Project
Real-time speaker de-identification and speech obfuscation system
Abstract
The increasing of eavesdroppers who use voice-based mass surveillance for collecting and analyzing user-generated audio data is raising concerns about security and privacy, particularly in the areas of speaker recognition and automatic speech recognition. Audio adversarial examples have been proposed as a way to fool these systems, but their usefulness, different image adversarial attacks extensively studied in the literature, is restricted in real-time scenarios. In addition, audio adversarial attacks need to target a phrase or sentence rather than a single label as in the adversarial image attacks. As a result, research on adversarial attacks in the audio domain is more challenging than in the image domain.
In this two-year proposal, we aim to develop attack systems that work as defenses capable of anonymizing users' identities and protecting users' speech sensitive information from eavesdroppers. Our attack systems are in the digital domain where attacks are passed to the victim model over a digital channel, thereby mitigating or removing conspicuous noise in adversarial examples. In the first year, we propose lightweight adversarial generators that are capable of producing inconspicuous perturbations while satisfying low latency constraints in streaming fashion, real-time on-device. Furthermore, in the second year, we focus on developing attacks with strong transferability that are easily transferred to other recognition systems without requiring retraining of the attack model for each new recognition system. This allows the attack to remain effective against unseen recognition systems, thereby increasing its practical value in a variety of settings. In this proposal, we will conduct extensive experiments and comparisons in terms of metrics such as perceptual study, word error rate, and character error rate to evaluate the efficiency of our systems.
Key Research Topic
Team Member
Research Fellow/ Prof. Chun-Shien Lu
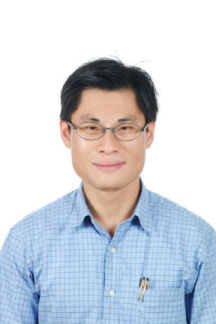
Contact
Research Fellow (Prof.) Chun-Shien Lu
Tel: +886-2-27883799 #1513
Email: [email protected]
Add: No 128, Academia Road, Section 2, Nankang, Taipei 11529, Taiwan